The value of a well-designed clinical registry with high-quality data cannot be overstated. Clinical registries are the most effective way to collect real-world data to provide real-world evidence for data-driven clinical practice, improvement of patient care, and to provide relevant data for research purposes. However, to be viable and become integrated into the busy daily workflow, registries must be easy to use and give valuable and relevant results to the contributors in the data collection process. Here, we provide advice and practical guidelines for designing high-quality clinical registries. Care should be taken to avoid common pitfalls that often undermine the best intentions.
1. Define the purpose
It is impossible to design a good registry without a clearly defined purpose. Collecting data across the board in a broad manner with a “more-the-better-to-be-on-the-safe-side” mentality is the route to designing something that will not be viable in real life. Without a clearly defined purpose, your colleagues and collaborators will lose interest in contributing. By not precisely defining the purpose or the hypothesis, the risk of collecting irrelevant parameters and missing significant ones is high.
A clinical registry can serve one single purpose or a combination of many. Improvement of care (clinical audit), research, health economics and resource management are the most common use cases in the hospital setting. Registry-based clinical trials (also called pragmatic trials) have become a more efficient path in medtech, biotech and pharma compared to costly and time-consuming conventional randomised clinical trials. Regulatory requirements for postmarket surveillance of medtech equipment have also emerged as an important use case.
Whether a registry is intended to serve a single purpose (i.e. clinical audit) or multiple purposes (i.e. clinical audit and secondary use for research) will, in most cases, have implications for the registry's design. It may also have regulatory implications related to requirements for consent from the data subjects, limitations and duration of data storage etc.
So, work your way backwards: What is your purpose, which questions do you want to be answered, or what hypothesis do you want to test? Then determine the specific parameters you need - not more, not less. And this brings us to the next point:
2. Be sparse with your parameters
Keep the number of parameters for registration as low as possible. The risk of missing data scattered throughout the dataset increases exponentially as the number of parameters increases. This is one of the most common pitfalls when designing a register. Down the road, this will make it hard to obtain all data on every entry (patient), which may result in an incomplete dataset with a lot of missing data that reduces the data quality and undermines statistical analyses.
Be critical of every new variable you create - all variables should be added on a need-to-know basis, not a nice-to-have basis. Consider whether the data will be difficult to find for the ones who will be entering the data, and weigh it against how important it is for the results and the registry’s purpose. When defining the outcomes (e.g. survival, cure, mortality or patient-reported outcomes such as quality of life), clearly define the explanatory variables you will need to draw valid conclusions and make sure that you have a clear understanding of the differences between association, correlation and causation. Also make sure that you understand the different designs of clinical studies if the registry will be used for research purposes.
To conclude, less is by far usually more, as a complete dataset means higher data quality, and the usefulness and the value of the registry increase as more solid conclusions can be drawn.
3. Use defined and standardised variables where possible
Choose variables and standards that are common to your field. Reporting using well-defined standards makes it possible to compare your results with other relevant datasets. Precise definitions of each variable must be available to the users of the register. This also applies if you use scales (such as not satisfied - satisfied - very satisfied), where the meaning of each value must be clearly defined.
4. Plan ahead for analysis
Whenever possible, choose structured data types (e.g. categorical, numerical or date). Unlike free text, these data types can be used directly for quantitative analysis and statistics. Free text should be reserved for supplementary information.
The design process should always include a data analysis plan. The data analysis plan should include both how you intend to present the data in text, graphs and tables, what statistical analyses you intend to perform and the required data you need to obtain valid results. Be practical, and it may be helpful to make a dummy of how you plan to present the data. If the registry will be used in weekly meetings, what are the relevant parameters, and how do you intend to present the data? Again, have a clear perception of your endpoints and your explanatory variables.
5. Be mindful of the context of data capture
When designing a clinical registry that is to be used within a clinical context, a key is to pay particular attention to how the data-entering procedure will fit into the everyday life of the healthcare professionals that will be working with the registry on a daily basis. Imagine the setting where data will be collected and registered into the database. Where is the person when entering the data? What information do they have available? Where is the information found? Is it obtained directly from the patients, in the patient records or other digital systems?
Create your data entry forms in a manner that matches the workflow of the person entering data. The smoother the registration process is, the more likely it is that busy clinicians will be able to complete the forms. Data that is not readily available in everyday work is likely to be missed.
6. Ensure value for all parties involved
Most registries involve multiple persons contributing to the data collection and registration process. Data may also be collected by automatic data capture through integration with other data sources, but in the majority of cases, at least some data is added manually. If multiple persons are involved in the manual registration process, it is good advice to make sure that there is “something in it” for everyone involved. Relevance and involvement give a higher value to the registry itself and translate to enthusiasm and engagement, and reduce the risk of missing data. For all data analyses, whether it involves statistical methods or not, missing data will undermine the data quality and may even render the registry unusable. Basic principles for registry design to avoid missing data must therefore be followed. Parameters that are not perceived as meaningful for the persons involved are likely to be ignored and should be excluded. So try to focus on parameters that are perceived as relevant and readily available.
7. Perform a “user test”
When you are done with creating the database and the entry forms, try it out in real life. Perform a user test with the persons who will register data and get their feedback. Remove or alter parameters that can be misinterpreted, and adjust the design if needed to ensure an optimal workflow in the data collection process.
To sum up, our best advice is to be selective and only include relevant parameters based on a clearly defined purpose and with an understanding of how the data will be analysed and presented. Keep the number of parameters as low as possible. Try only to include standardised parameters that are readily available and pay attention to how the data-entering procedure will fit into the everyday life of the healthcare professionals that will be working with the registry. Make sure there is “something in it” for everyone involved in the data registration process to ensure engagement, relevance and value.
More From Ledidi Academy
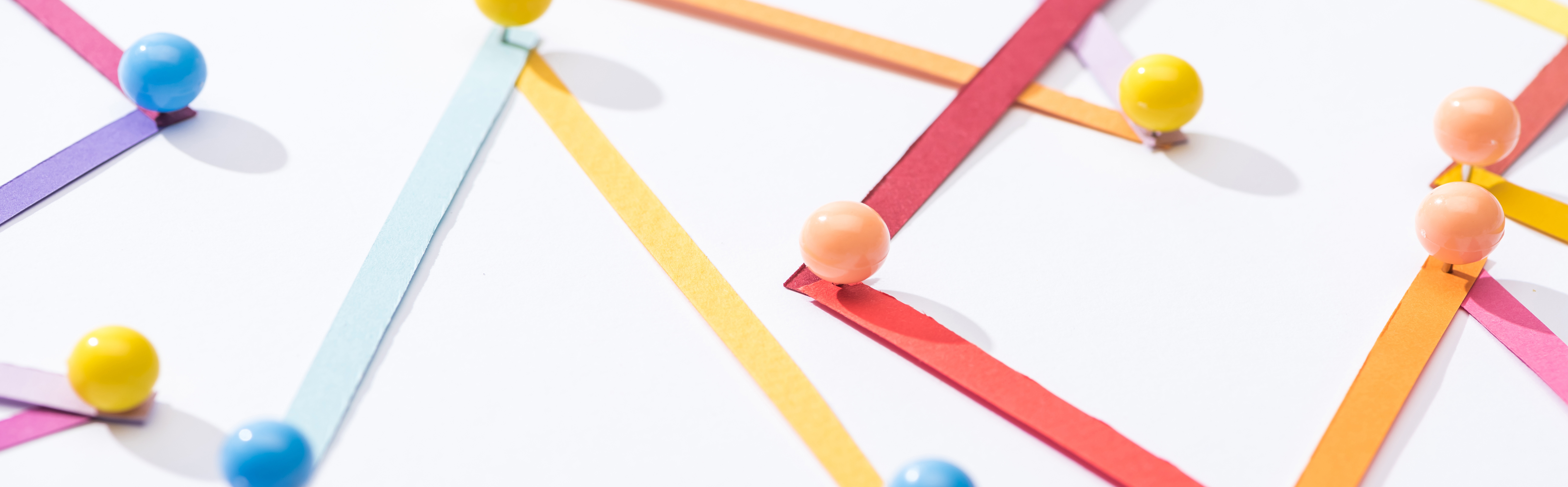
The difference between association, correlation and causation
- Statistical analysis
- Tips and insights